Table of Contents
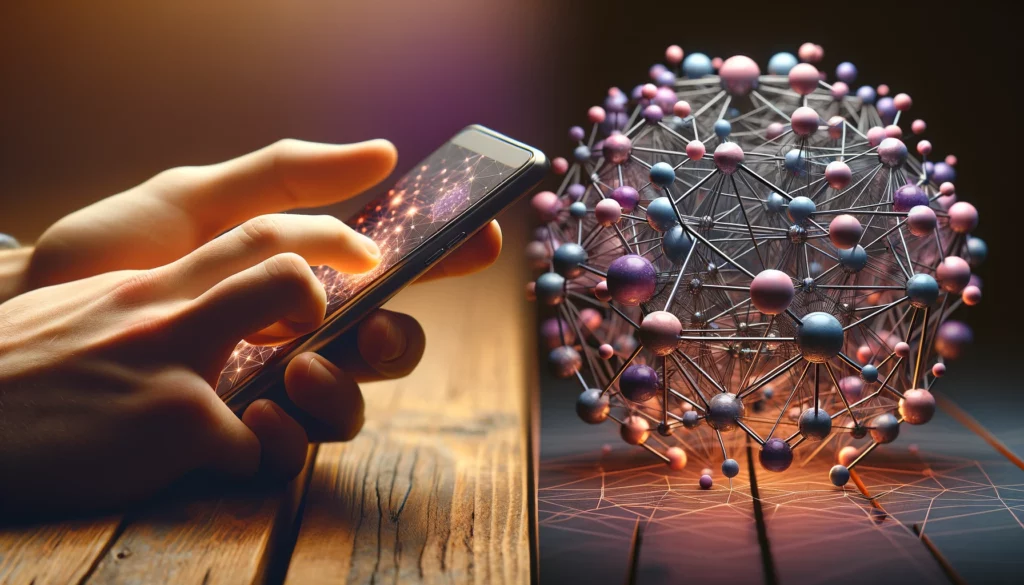
How is generative AI used in content creation? Present and future concerns
Generative AI is revolutionizing content creation across digital platforms, offering tools that automate and enhance the production of text, images, and videos. This technology not only streamlines workflows but also personalizes user experiences through sophisticated algorithms. However, as generative AI continues to evolve, it raises significant concerns regarding the authenticity of content and the potential spread of misinformation. The challenge lies in developing and implementing effective detection methods to distinguish between human and AI-generated content, ensuring integrity and trust in digital media as we move forward.
How AI impacts on social media
Artificial intelligence (AI) significantly shapes social media by streamlining content creation and management, which enhances user engagement and personalizes experiences. However, it also introduces challenges such as the proliferation of fake news, deepfakes, and sophisticated misinformation, making it difficult for users to distinguish between authentic and AI-generated content. This downside can appear because of creators who fail to verify the veracity of content, but also ill-intentioned users who benefit from these tools for their wrong-doings. While AI detectors aim to address these issues, they often struggle to keep pace with the evolving capabilities of AI, resulting in frequent inaccuracies. In this discussion, we will explore more effective solutions for identifying AI-generated content, thereby enhancing the safety and reliability of social media platforms. This is crucial for understanding how AI is being used on social media and for developing strategies to mitigate its risks.
How do AI content detectors work (and their reliability)
AI content detectors employ algorithms to analyze linguistic and stylistic features, aiming to identify patterns typical of AI-generated text. Despite the availability of various online tools designed for this purpose, their reliability is often questionable. These detectors struggle to distinguish between AI-generated and human-authored content accurately due to the rapid advancement of AI technology, leading to frequent false positives and the inability to adapt quickly to new AI models. Even if most of these detectors are able to determine if the content is human or AI-generated, many of them fail to state what percentage of the content is human or AI. Have a look at this experiment carried out by Jeff Bullas, where he fed an AI detector with both human and AI-generated content, and he got poorly refined results, having his own text scored as ”32% human”.
Challenges in detecting AI-generated content
Detecting AI-generated content presents numerous challenges as technology evolves. Advancements in AI complicate the identification process, and the lack of clear indicators makes differentiation tough. Additionally, the consequences of incorrect detections can have significant implications, underscoring the complexity of effectively identifying artificial content.
Advancements in AI technology
The rapid advancements in AI technology significantly complicate the detection of AI-generated content. As AI models become more sophisticated, they produce outputs that closely mimic human writing styles and behaviors. This continuous improvement blurs the lines between human and machine-created content, making traditional detection methods less effective and requiring constant updates to detection algorithms.
Lack of clear indicators
AI-generated content often lacks clear, definitive indicators that reliably distinguish it from human-created material. The subtleties in text, image, or video created by advanced AI can mirror human nuances closely, making it challenging for detectors to pinpoint artificial origins. This absence of reliable markers demands more sophisticated analytical tools and approaches, which can analyze deeper patterns and contextual discrepancies.
Consequences of incorrect detections
Incorrect detections of AI-generated content can lead to several adverse consequences. False positives, where human-created content is misidentified as AI-generated, can unjustly restrict or penalize creators, affecting their credibility and visibility. Conversely, false negatives allow AI-generated misinformation to proliferate unchecked, potentially spreading falsehoods and influencing public opinion under the guise of authenticity.
How to detect AI content: Most effective techniques
Identifying AI-generated content accurately requires adopting the most effective detection techniques. These include sophisticated text, image, and video analysis methods, which leverage advanced algorithms and deep learning models. This section explores the most reliable techniques for discerning AI-created materials, ensuring higher accuracy and minimizing false detections.
Text-based detection methods
Text-based detection methods are crucial for discerning between human-typed and AI-generated text. These methods employ Natural Language Processing (NLP) algorithms, linguistic analysis, and sentiment analysis to detect subtle anomalies and patterns that may suggest AI authorship. Enhancing these techniques, for example, by implementing a keyboard SDK into your company apps, is vital for ensuring authenticity in digital communication, providing a robust tool to safeguard against the infiltration of AI-generated content.
Natural Language Processing (NLP) algorithms
Natural Language Processing (NLP) algorithms analyze the structure and composition of text to detect AI-generated content. By examining syntactic patterns, grammar, and word usage, NLP algorithms can identify patterns that typically do not occur in human writing. This method is particularly effective in recognizing content that lacks the understanding of language nuances and context that human authors usually exhibit.
Linguistic analysis to identify anomalies
Linguistic analysis focuses on the deeper aspects of language use, such as semantics, coherence, and the stylistic choices unique to human authors. This technique scrutinizes text for anomalies that might indicate AI generation, such as unusual phrasing or inconsistent tone. Linguistic analysis helps distinguish AI content by pinpointing elements that do not conform to typical human linguistic patterns.
Sentiment analysis to detect patterns
Sentiment analysis involves evaluating the emotional tone behind words to identify AI-generated text. AI often struggles to accurately replicate the subtle emotional nuances conveyed in human writing, making this method effective for detection. By analyzing sentiment consistency and relevance within the context, this approach can highlight discrepancies and unnatural patterns that suggest the presence of AI-generated content.
Image-based detection methods
Image-based detection methods utilize advanced algorithms to analyze visual content for signs of AI manipulation. These techniques are critical in identifying doctored images, deepfakes, and other forms of synthetic media. Employing reverse image searches, image manipulation detection, and deep learning models, these methods help to ensure the authenticity of images circulating on social media and other platforms.
Reverse image search algorithms
Reverse image search algorithms help detect AI-generated or manipulated images by comparing them against vast databases of known images. When an image is uploaded, these algorithms scan through countless online sources to find matches or similar images. This method is effective in identifying images that have been stolen, manipulated, or reused from other contexts, thus signaling possible AI involvement.
Image manipulation detection techniques
Image manipulation detection techniques focus on uncovering alterations in images, such as inconsistencies in lighting, shadows, or edges that may suggest digital tampering. These techniques analyze the image’s metadata and pixel-level details to detect anomalies that are often signs of AI manipulations like deepfakes or photoshopped images.
Deep learning models for image analysis
Deep learning models for image analysis are highly sophisticated and capable of detecting subtle and complex patterns that indicate AI involvement. These models are trained on large datasets of real and AI-generated images, learning to discern between genuine human-created images and those altered or created by AI. Their ability to recognize minute discrepancies in texture, color gradation, and form makes them invaluable in the fight against AI-generated visual misinformation.
Video-based detection methods
Video-based detection methods are essential for identifying AI-generated or manipulated video content. These methods use sophisticated techniques to analyze both visual and auditory elements of videos, including frame analysis, audio analysis, and deep learning models. By scrutinizing these aspects, the methods help confirm the authenticity of video content and detect synthetic alterations.
Frame analysis for anomalies
Frame analysis involves examining individual frames of a video for irregularities that might indicate manipulation. This method checks for inconsistencies in visual continuity, such as abrupt changes in lighting, background, or subject appearance that are not typically present in authentic footage. Such anomalies can often suggest the presence of edited or entirely AI-generated video segments.
Audio analysis for inconsistencies
Audio analysis focuses on detecting inconsistencies in the sound of a video that might indicate manipulation. This technique examines aspects like voice continuity, background noise levels, and sound quality that may not align with the visual components of the video. Discrepancies in audio can be a strong indicator of tampering or the use of AI technologies such as voice synthesis.
Deep learning models for video content analysis
Deep learning models for video content analysis are highly effective in distinguishing between genuine and AI-manipulated videos. These models are trained on extensive datasets featuring both real and synthetic videos, enabling them to identify subtle patterns and anomalies that human reviewers might miss. Their comprehensive analysis covers everything from facial expressions and movement dynamics to the coherence of audio-visual elements, providing a robust defense against AI-generated video content.
Our final thoughts
In conclusion, the rapid evolution of AI and the corresponding advancements in AI detection present a complex landscape for content creators and consumers alike. AI brings undeniable benefits by enhancing the efficiency and reach of content across digital platforms. However, it also poses significant risks, particularly in the spread of misinformation through AI-generated content. This duality underscores the need for robust mechanisms to verify content authenticity. Among the emerging solutions, keyboard typing detection stands out as a promising method to ascertain whether content is human-authored or AI-generated. This technology can play a crucial role in maintaining the integrity of digital communication.
As we navigate this evolving digital landscape, we encourage you to explore innovative tools such as the Fleksy virtual keyboard. Fleksy is at the forefront of integrating AI detection capabilities, offering users not just enhanced typing experiences with keyboard solutions for industries but also a layer of security against AI-generated content.
Discover how Fleksy can transform your digital interactions by ensuring that what you read and engage with is genuine, among the many benefits of virtual keyboards. Embrace the future of digital content with Fleksy, where technology meets trust.
FAQs
How to detect AI writing?
Detecting AI writing involves analyzing text for unusual patterns and anomalies that are not typical in human writing. Keyboard-based solutions, like advanced algorithms integrated into virtual keyboards, can track typing patterns and rhythms to help determine if the content is generated by a human or an AI, enhancing the detection process.
Can AI-generated text be detected?
Yes, AI-generated text can be detected using various methods, including linguistic and stylistic analysis. Keyboards that monitor typing behavior offer a unique approach, as they can identify the natural cadence and errors typical of human typing, which are often absent in AI-generated text.
Can Instagram detect AI-generated images?
Instagram and other social media platforms are increasingly utilizing AI to detect and manage AI-generated images. They employ image analysis techniques to spot inconsistencies typical of generated content. Incorporating these capabilities into the platform’s interface, possibly through extensions or integrated tools within the app, could further enhance detection accuracy.